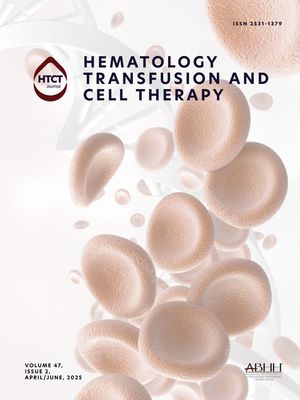
Plasma cells (PC), derived from B lymphocytes, are crucial for the immune response due to their ability to secrete antibodies. Understanding their growth and dynamics is essential, especially in the contexts of autoimmune diseases and cancers like multiple myeloma (MM), where abnormal proliferation of PC can have severe repercussions. Analyzing PC growth through mathematical (Math) models helps in elucidating these processes and developing targeted treatments (TT). Basic Math Models of Cellular Growth is often modeled using differential equations, with the exponential and logistic models being particularly prominent. Exponential Model (EM): represents unrestrained growth and is described by N(t)=N0ertN(t) = N_0 ê{rt}N(t)=N0ert, where N(t)N(t)N(t) is the cell population at time ttt, N0N_0N0 is the initial population, and rrr is the growth rate. This model is applicable to PC in early stages or environments without constraints, such as initial in vitro studies. Logistic Model (LM): Unlike the EM, the LM accounts for environmental limits. It is expressed as N(t)=KN0ertK+N0(ert-1)N(t) = frac{K N_0 ê{rt}}{K + N_0 (ê{rt} - 1)}N(t)=K+N0(ert-1)KN0ert, where KKK denotes the carrying capacity. This model is more realistic for describing PC proliferation in tissues with limited space and resources, reflecting a more controlled growth environment. Stochastic Models (SM): When variability and uncertainty play significant roles, SM become crucial. These models incorporate randomness, representing fluctuations in cell behavior due to both intrinsic and extrinsic factors. Such models account for time-dependent variations in these probabilities, offering a dynamic view of PC behavior that reflects real-life biological variability and environmental influences. Compartmental models: Divide the PC population into distinct states, such as resting, proliferating, and apoptotic cells. These transitions are governed by differential equations describing the rates of change between compartments. These models are valuable for understanding the dynamics of PC during immune responses or pathological conditions. For example, in MM, compartmental models can help illustrate how disruptions in PC regulation contribute to disease progression. Math models are increasingly used in clinical settings to predict PC behavior in response TT. By simulating the effects of immunomodulatory therapies or chemotherapy, these models assist in evaluating and optimizing TT strategies. Modeling the interaction between PC and their microenvironment, such as the impact of cytokines, is crucial. This approach helps in understanding how environmental factors support or inhibit abnormal PC growth, which is vital in diseases like MM. Modeling PC growth involves challenges such as cellular heterogeneity and the complexity of the tumor or inflammatory microenvironment. Advances in bioinformatics and computational technologies are facilitating the development of more sophisticated models that integrate high-throughput data, including genetic sequencing and gene expression profiles.
ConclusionMath models of PC growth provide a powerful framework for exploring cellular dynamics and developing TT strategies. Despite significant progress, the complexity of cellular behavior and environmental interactions presents ongoing challenges. Future advancements will likely rely on increasingly integrative and data-driven approaches to enhance our understanding and management of PC-diseases.