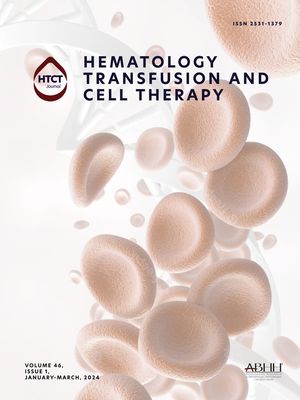
The prediction of Recurrent Venous Thromboembolism (RVTE) plays a crucial role in enhancing the prognosis of patients with coagulation disorders. In recent times, there has been a notable surge in studies focusing on developing scores based on machine learning models that utilize clinical data and blood counts as input. However, due to the inherent black-box nature of machine learning models, understanding the relationship between model features and the phenomenological aspects associated with blood clot formation and thrombosis has been challenging. To address this, incorporating mathematical modeling of blood coagulation alongside prediction techniques presents an alternative approach to improve both prediction accuracy and our understanding of the underlying phenomenological aspects.
AimsThis study aimed to propose a patient-specific spatiotemporal phenomenological model for clot formation and utilize it to predict RVTE.
MethodsA spatiotemporal model for blood clot formation was presented, encompassing convective, diffusive, reactive, and platelet aggregation effects. Blood flow was accurately modeled using Navier-Stokes equations, incorporating an additional term for flow resistance due to blood clot formation. The model included quantitative variables from a complete blood count, enabling simulation personalized to each patient. The finite volume method was employed to solve the model within a clotting time of 10 minutes. The simulations involved 235 patients who had a thrombotic event, monitored by the State University of Campinas Blood Center, who underwent routine blood tests. Out of these patients, 49 were diagnosed with RVTE. Principal Components Analysis (PCA) and Partial Least Square (PLS) regression were utilized to identify the key variables influencing clot formation. These critical variables were then employed as inputs for an Artificial Neural Network (ANN) model, facilitating binary classification for RVTE prediction.
ResultsThe patient-specific model demonstrated high sensitivity in distinguishing clot size variations between patients, with thrombus size directly related to platelet count in blood plasma. However, relying solely on clot size for RVTE prediction yielded low accuracy. To address this, the proposed strategy of using PCA and PLS to identify critical points in space and time successfully reduced the number of significant variables to 20. This reduction was essential in making the ANN training feasible, as the high dimensionality of the original dataset would otherwise result in excessive computational costs. The optimized ANN model achieved promising results: an Area Under Curve value of 81.51%, accuracy of 86.81%, and a true-positive ratio of 79.59%.
DiscussionThe findings suggest that incorporating phenomenological modeling based on blood test parameters can generate distinct clot formation profiles for individual patients, revealing differences in shape, growth time, and size. Dimensionality reduction techniques, such as PCA and PLS, effectively extract critical features, enabling the ANN-based model to achieve high accuracy in RVTE prediction.
ConclusionsIntegrating phenomenological modeling, machine learning, and statistical tools holds great promise for RVTE prediction. With further validation, this model could find application in clinical practice, providing valuable insights into blood clot modeling for patients at risk of RVTE.