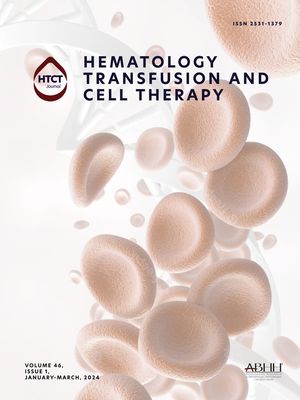
Mathematical models play a crucial role in comprehending the intricate interactions and cascade reactions of chemical species involved in the blood coagulation cascade. Accurate models facilitate the prediction of thrombin profiles, aiding in diagnosing and prognosis diseases like Venous Thromboembolism (VTE). However, current mathematical models in the literature primarily rely on the concentration of chemical species and kinetic constants, overlooking essential variables such as age, sex, smoking, obesity, and diseases known as risk factors for thrombosis.
AimsThe primary objective of this study is to develop a novel model where the kinetic parameters of the coagulation cascade are dependent on clinical and blood count data. This approach generate patient-specific thrombin profiles, considering well-known risk factors for thrombosis.
MethodsA sensitivity analysis was performed on a coagulation cascade model based on a first-order differential equation system of 44 kinetic parameters. Critical kinetic parameters were allowed to vary between 30% and 300% of their default value. A hybrid Artificial Neural Network (ANN) model was employed to adjust these kinetic parameters, utilizing clinical and blood count data from 235 patients, of which 49 had thrombosis. The structure of the proposed hybrid model consists of an input layer using the clinical and blood count data of the patients, two intermediate layers, and a layer of prediction of the values of the critical kinetic parameters, which are used to calculate the thrombin profile from the mathematical model of the coagulation cascade. The ANN was optimized using a genetic algorithm to adjust weights and bias. The goal was to ensure that patients with thrombosis had a substantial area under the thrombin curve over time, while patients without thrombosis exhibited a lower area under the thrombin curve.
ResultsThe analysis identified eight highly sensitive kinetic parameters related to factor V activation and thrombin-antithrombin III complex formation. These parameters were influenced by all input variables, especially age, sex, obesity, anticoagulant use, smoking, factor V Leiden, glucose, triglycerides, factor VIII, and G20210A mutation. The hybrid ANN model successfully predicted VTE recurrence with an accuracy of 98.72% and an Area Under Curve of 99.41%.
DiscussionThe findings of this model align with existing literature on the relationships between input variables, kinetic parameters, and thrombosis risk. The adopted approach allowed for the generation of patient-specific thrombin curves, which hold great potential for patient-specific models. The genetic algorithm proved efficient for optimizing the ANN hybrid model, achieving remarkable prediction accuracy.
ConclusionsMachine learning techniques have demonstrated their capability to enhance models, especially in scenarios where the underlying processes are complex or not fully understood. Incorporating VTE risk factors into the cascade model enhances blood clotting models and improves their generality. This methodology could be employed with further validation to identify new therapeutic targets for VTE treatment.