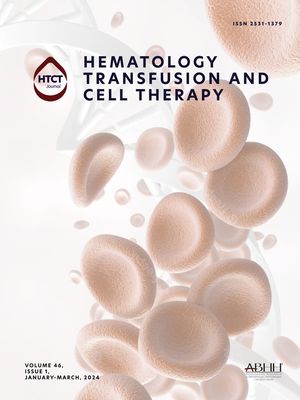
Current blood clot formation models often overlook the influence of risk factors on thrombus formation, and they assume constant kinetic parameters for the coagulation cascade, leading to identical thrombin production profiles for all individuals. However, in reality, each person exhibits a unique thrombin production pattern. To address this limitation, developing patient-specific mathematical models based on easily obtainable parameters is crucial. Representing the blood clot growth in terms of quantitative variables from complete blood count data provides valuable insights.
AimsThe primary goal is to present a novel spatiotemporal hybrid model for thrombus formation that combines phenomenological aspects with an adjusted coagulation cascade using artificial intelligence. Based on clinical and complete blood count data, the model aims to differentiate thrombus formation between patients with and without Recurrent Venous Thromboembolism (RVTE).
MethodsThe proposed model encompasses convective, reactive, and diffusive effects occurring in vivo during clot formation. To regulate the chemical reactions of the coagulation cascade, kinetic parameters were adjusted based on easily measurable variables such as age, sex, obesity, smoking, and blood count parameters. The numerical grid was solved using the finite volume method. The simulations were conducted on 235 patients from the blood center of the State University of Campinas. The clot size was normalized between 0 and 1, and an Artificial Neural Network (ANN) model was employed to predict RVTE based on this input.
ResultsBy comparing thrombus size, platelet count, and RVTE outcomes for simulations with and without artificial intelligence adjustment, it was observed that the adjusted model demonstrated superior separation of outcomes. The p-value of thrombi size between the two models was 0.0492, indicating a significant impact of kinetic parameter adjustment on thrombus size. The binary classifier of the ANN achieved an accuracy of only 78% without kinetic adjustment, whereas the proposed model with adjusted kinetic parameters reached an accuracy of 98.3%.
DiscussionAltering the kinetic parameters of the coagulation cascade model considerably affected the size of the blood clot generated by the phenomenological model. The ability to adjust these parameters based on easily obtainable data enables the individualization of thrombus profiles for each patient, leading to a hybrid and specific model for every individual. This individualization significantly improved the distinction of clot size for different output classes (with/without RVTE), substantially enhancing the accuracy of the binary predictor.
ConclusionsThe adjustment of kinetic parameters allowed for personalized thrombus formation modeling for each patient. Future validations can improve mathematical models for patients at risk of RVTE, contributing to better prevention and treatment strategies.