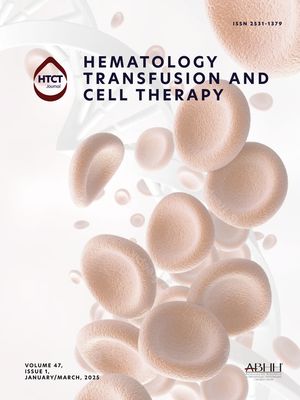
Unsupervised machine learning techniques are employed to understand patterns and behaviors of variables in databases. Multiple Correspondence Analysis (MCA), an extension of correspondence analysis, can be used to verify the association of categorical variables and their categories. In this study, we evaluated the relationship between clinical-demographic variables of 154 patients with nodal peripheral T-cell lymphoma (PTCL) to understand how these variables relate to the unfavorable clinical outcome, death.
MethodologyMCA was used to reduce the dimensionality of the real world database by creating two dimensions, 1 and 2, which were subsequently categorized into group 1 and group 2. Dimension 1, comprising ECOG, IPI, treatment, overall response, remission, and bone marrow transplant, represented about 30% of the total observed variance. Survival analysis was conducted to assess the association between these groups and overall survival (OS) and mortality rate.
ResultsThe categories of dimension 1, group 1 and group 2, were associated with OS and mortality rate, with group 1 being associated with 5.5 months (95% CI: 2.70 – 8.40) of OS, while group 2 had a survival time of 277.20 months (95% CI: 91.21 – 463.12). Moreover, the mortality rate in group 1 was 87% (n = 67) and in group 2 it was 36% (n = 28) (p < 0.001). The risk of death for group 1 was 11.11 times (95% CI: 9.75-18.30; β= 2.41; p < 0.001).
DiscussionThese findings indicate a significant disparity in survival outcomes based on the clinical-demographic profiles of the patients. Group 1, characterized by poorer clinical indicators, exhibited substantially shorter OS and higher mortality rates. The ability of MCA to effectively reduce dimensionality while preserving the clinical relevance of the variables underscores its utility in identifying key prognostic factors.
ConclusionThe MCA technique was effective in reducing the dimensionality of the database, maintaining the clinical characteristics of the variables robustly for use in Cox regression. This approach can aid in the identification of high-risk patient groups and inform treatment strategies aimed at improving clinical outcomes.