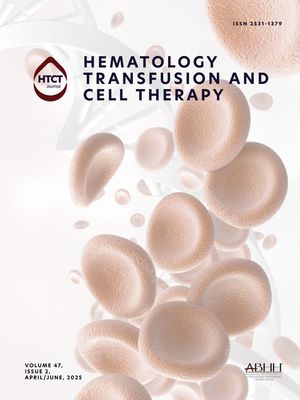
Computed tomography (CT) scans are integral to cancer patient diagnosis, revealing changes in body composition linked to survival progression. The conventional approach to body composition analysis using CT scans is labor-intensive and expensive, demanding skilled professionals and licensed software for manual segmentation of Regions of Interest (ROIs). To address these challenges, we introduce a Deep Learning algorithm designed for automated CT image segmentation, presenting an efficient alternative that overcomes the limitations of the current methodology. Beyond the advantages of speed, automation enhances result uniformity and enables uncertainty estimation. In this presentation, we will show preliminary results from our algorithm, highlighting its potential contributions to survival analysis in cancer patients.
ObjectivesThe primary goal of this study was to develop an automated segmentation algorithm for CT scans using Deep Learning models.
Materials and MethodsIn developing segmentation algorithms, a dataset of 453 CT slices at the L3 lumbar vertebral level from gastric cancer patients was utilized, with an 80% training and 20% testing partition. Employing the UNET+ResNet18 deep learning architecture, supervised training utilized manually generated segmentation masks as references. Four dedicated UNET+ResNet18 algorithms were trained for distinct ROIs: Skeletal Muscle (SM), Intramuscular Adipose Tissue (IMAT), Visceral Adipose Tissue (VAT), and Subcutaneous Adipose Tissue (SAT). Segmentation performance on the test set was evaluated using the Dice Coefficient, underestimation and overestimation percentages, Bland-Altman analyses, and qualitative visual inspection of segmented images.
ResultsThe UNet+ResNet18 models demonstrated superior segmentation performance for SM, VAT, and SAT, achieving mean Dice scores exceeding 0.95. In comparison to manual segmentation, the Deep Learning algorithm exhibited minor average underestimations and overestimations, both below 5% for these tissues. However, IMAT segmentation exhibited relatively lower performance, with a mean Dice score of approximately 0.86 and underestimation and overestimation percentages around 15% and 13%, respectively. The Bland-Altman analysis revealed mean bias and limits of agreement for mean radiodensities of SM, VAT, SAT, and IMAT as follows: 0.14 [-0.82, 1.10] HU, -0.53 [-2.03, 0.98] HU, -0.18 [-1.70, 1.33] HU, and 0.48 [-3.86, 4.82] HU, respectively.
ConclusionThe Deep Learning approach provides a standard and fast solution for CT image segmentation, demonstrating good results for SM, VAT and SAT. For these tissues, derived radiomics features could provide valuable insights into the analysis of cancer patient outcomes. Further studies are necessary for enhancing IMAT segmentation, given its challenging small area. Additionally, future investigations should focus on uncertainty estimation in CT images, exploring its impact on segmentation procedures and radiomic feature extraction.