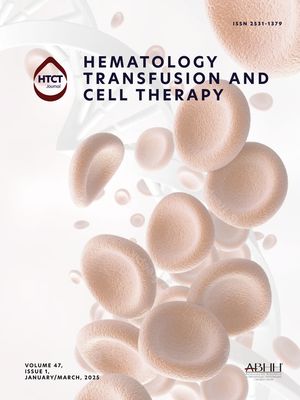
Idiopathic multicentric Castleman's disease (iMCD) is an ultra rare lymphoproliferative disorder difficult to diagnose due to its rarity (prevalence of 6.9-9.7 cases per million in the United States) and the lack of awareness among physicians. Median time between symptoms onset and the diagnosis of iMCD in Brazil is estimated to be 18 months. We propose that an artificial intelligence (AI)-based algorithm working on electronic health records may help to identify patients suspected of Castleman disease that were missed during medical evaluation.
MethodsFirstly, symptoms listed in the CDCN iMCD criteria [Fajgenbaum DC, et al. Blood. 2017;129(12):1646-57] were defined by medical terms. Medical definition contained ICD - 10 codes, lab test results and phrases that may be found in the medical texts (descriptions of imaging tests, interviews, epicrisis). SPACY models with in house developed regular expressions (regex) were used to identify phrases (entities) assigned to symptoms in the unstructured medical texts. Entity context defined as negation, family relation, historical or hypothetical event was determined by the BERTH model that went through additional training on the Saventic database. Patients were ranked based on the symptoms they possessed. Patients were ranked only when lymphadenopathy was identified and patients already diagnosed with iMCD (four cases) were excluded. Physicians with expertise in iMCD led the review of patients’ charts with the highest score.
Results594 953 patients'charts from a tertiary Brazilian hospital were screened by the algorithm and iMCD clinical and/or laboratory features were identified in 102 patients, which had higher probability of having iMCD. Of those, 12 had mimicker conditions with overlapping iMCD features and three others required further assessment.
ConclusionThis AI driven tool was able to identify patients who have iMCD features. Screening with this tool found at least three candidates for additional evaluation. In the future, algorithms like the one we present may facilitate screening for iMCD.
Conflicts of interestAAGSB, DL: Saventic Health (honoraria). MK, WA, GB, KL, MJD, MD: Saventic Health (current employment).