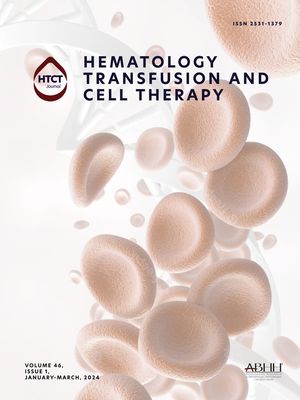
KMT2A (MLL) gene rearrangements (KMT2A -r) are associated with diverse acute leukemias. Given the high number of KMT2A fusions, screening methods guide their detection regardless of partner gene. For instance, immunophenotyping using an anti-CSPG4 (NG2) antibody or split-signal fluorescence in situ hybridization (FISH) are routinely used for the identification of KMT2A -r. However, we recently revealed that certain KMT2A fusions (e.g. KMT2A-USP2) might be underestimated because tradicional methods often fail to detect them. Therefore, complementary methods are needed for appropriate detection of KMT2A -r. In addition, the standard treatment of KMT2A -r acute leukemias has not significantly improved outcomes, despite many years of international efforts. Thus, the urgent need for novel therapeutic strategies is unquestionable.
ObjectivesHere we use a machine-learning model to unravel the most appropriate markers for prediction of KMT2A -r in all types of acute leukemia: AML, B-ALL, T-ALL, and ALAL. We also apply differential expression analysis using RNA-seq data to point out new therapeutic possibilities for KMT2A -r leukemia.
MethodologyOur analyses were performed on gene expression data from the TARGET project (n = 706; 73 KMT2A -r and 633 KMT2A -WT). We applied the BorutaSHAP variable selection to identify relevant predictors of KMT2A -r. After dividing our dataset into training (70%) and testing (30%) cohorts, a random forest model was trained for estimating KMT2A -r. Then, we performed an external validation in two independent cohorts: AML samples (n = 151) from the TCGA database, and ALL samples (n = 176) from the AP-HP, France. For the investigation of therapeutic targets and new drugs for KMT2A -r patients, we identified differentially expressed genes using RNA-seq data. Then, we performed pathway enrichment analysis and selection of candidate drugs using the Drug-Gene Interaction database, and overlapped results with the Genomics of Drug Sensitivity in Cancer database.
ResultsOur results revealed a set of 25 genes capable of accurately estimating KMT2A -r. They were significantly enriched in transcriptional misregulation in cancer (FDR ≤ 0.05). The oversampling method had the best combination of AUC, accuracy, sensitivity and specificity (0.931, 0.943, 0.917, 0.947, respectively) for estimating KMT2A -r. In other words, our model was able to predict 22 of 24 KMT2A -r patients and 178 of 188 KMT2A -WT patients. In the validation analyses, our model was able to correctly predict all eight KMT2A -r in AML (sensitivity = 1.000), but misclassified five of 11 KMT2A -r in ALL (sensitivity = 0.545). The LPAR3, SKIDA1, and MEIS1 overexpression were best associated with KMT2A -r compared to CSPG4 (NG 2), regardless of the type of acute leukemia. Of importance, high expression levels of LPAR3 estimated the occurrence of all KMT2A-USP2 fusions. Also, we identified 116 up-regulated genes in relapsed KMT2A -r acute leukemia, and 56 drug interactions with 13 genes. The KMT2A -r cell lines were more sensitive to foretinib treatment (Wilcoxon, p = 0.019) compared to KMT2A -WT.
ConclusionsIn this study, we observed that our algorithm provided highly accurate predictions for KMT2A -r acute leukemias. Therefore, we provide valuable options for screening KMT2A -r in leukemia, including LPAR3, SKIDA1, and MEIS1 overexpression. We also reveal that foretinib should be further investigated as a therapeutic alternative in KMT2A -r acute leukemia.