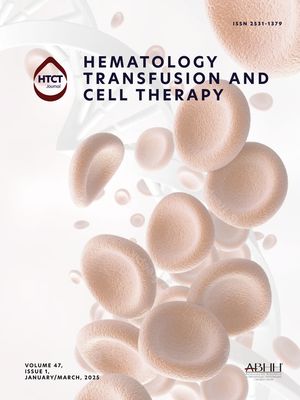
Prognostic models like the IPSS-R play a crucial role in assessing outcomes for patients with myelodysplastic neoplasms (MDS). However, recent advancements in machine learning (ML) offer the potential to uncover novel predictive variables and enhance prognostic accuracy. Models like ElasticNet are particularly adept at handling multidimensional data, thereby expanding the scope beyond the variables considered in IPSS-R.
ObjectivesAssessing the performance of ML in predicting overall survival in MDS patients by incorporating clinical and hematological variables not traditionally included in prognostic models.
MethodsWe conducted a retrospective cohort study at a single reference center involving patients diagnosed with MDS between 2004 and 2024. We included patients with available clinical outcomes, missing data was handled using the ’cart’ multiple imputation, following confirmation of non-random missingness through Little's test. The dataset was then randomly split into a training group (70%) and a testing group (30%). Utilizing group elastic net machine learning, an artificial intelligence model capable of selecting relevant variables and assessing their discriminative power, we constructed 3 receiver operating characteristic (ROC) curves to predict 1, 3, and 5-year survival, extracting the area under the curve (AUC) and identifying variables with non-zero coefficients. Based on these coefficients, we categorized our dataset into “High Risk” and “Low Risk” groups. Subsequently, we conducted a multivariate Cox proportional hazard regression analysis, adjusting for the new risk variable, age at diagnosis, sex, and transfusion burden. All statistical analyses were performed using R, with the involvement of packages such as ‘mice’, ‘gpreg’, ‘gplasso’, and ‘survfit’.
Results162 patients were included in this study. Using the group ElasticNet model, we identified 10 critical variables with notable predictive power: hemoglobin count, mean corpuscular volume, platelet count, presence of dysgranulopoiesis, presence of dysmegakaryopoiesis, serum iron, transferrin saturation, bone marrow cellularity, percentage of blasts in bone marrow, and percentage of ring sideroblasts. ROC curve analysis utilizing these variables'coefficients yielded AUCs of 0.863, 0.822, and 0.719 for predicting 1-year, 3-year, and 5-year survival, respectively. The coefficients were then extracted and used for risk stratification. 5-year survival rates were 24.3% for the High Risk group and 70.4% for the Low Risk group (p < 0.001, log-rank test). In multivariable Cox regression analysis, the risk group variable was the most discriminative predictor (HR = 3.56, p < 0.001), with sex, age at diagnosis, and transfusion burden also being significant (p = 0.01, 0.009, and 0.002, respectively).
DiscussionThe strong performance of the model, as evidenced by the ROC curve analysis, suggests that the selected variables offer substantial discriminative power. ML models offer more refined risk stratification than traditional methods, which may be useful in identifying occult relationships between variables. Validation in independent datasets may be necessary to strengthen the relationships herein exhibited.
ConclusionML is a valuable tool for risk classification and survival prediction, offering significant insights for clinical decision-making and patient management that may be overlooked by other methods.